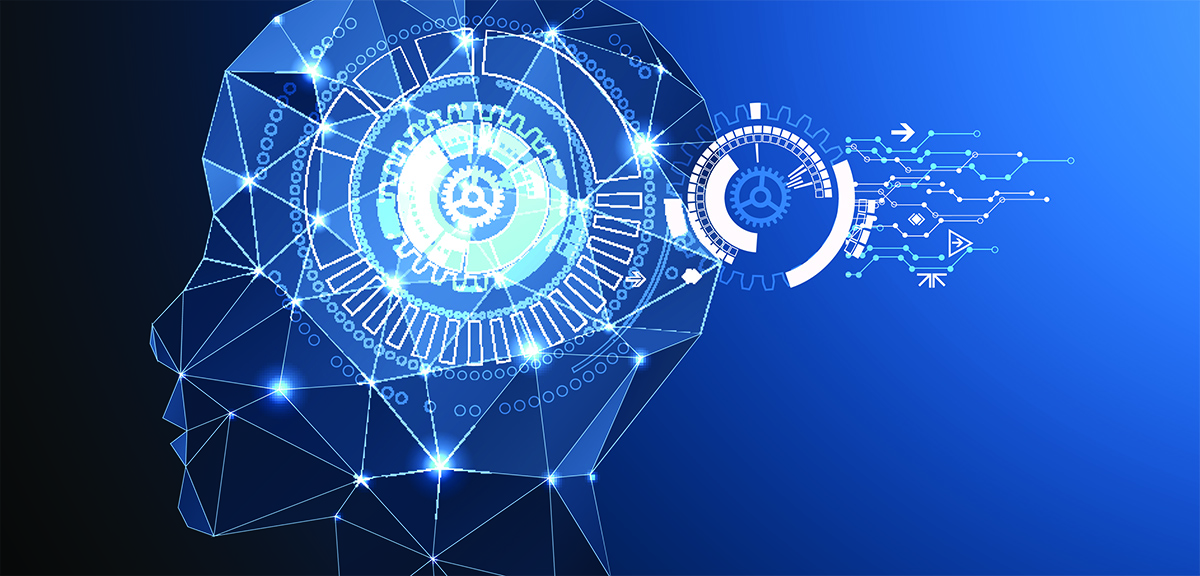
Dave Egts is chief technologist, North America public sector at Red Hat.
Artificial intelligence showed a lot of promise decades ago when many thought expert systems and fuzzy logic would be used everywhere. Unfortunately, that didn’t quite come to fruition, largely because the concepts were ahead of their time.
Today, AI is a reality. We use it at home (the aforementioned Alexa, or Apple’s Siri) and at work (so-called smart machines that do everything from monitoring social media traffic to providing second opinions for cancer treatments).
This proliferation is thanks to complementary enabling big data analysis, exa-scale storage, and cloud technologies that cost effectively assist AI algorithms with highly scalable methods to quickly access and analyze massive data sets.
As AI technologies become more and more popular, startups are getting acquired and integrated as software-as-a-service offerings from a small number of large companies like Google, Salesforce, IBM and others.
And as AI moves to SaaS, some government agencies and workloads may miss out on the benefits because of security and privacy requirements, which may put the government at a disadvantage compared to commercial counterparts and consumers.
For instance, many commercial and government IT enterprises take advantage of predictive risk analytics like NetApp’s AutoSupport, Red Hat Insights and others. By using these technologies, operations teams can get ahead of outages and security vulnerabilities even before their security teams are aware of problems. That’s great for commercial entities, consumers and government agencies with workloads at lower Federal Information Security Management Act levels.
Sadly, agencies with classified or sensitive workloads can’t use these services because their security requirements prohibit them from using the public cloud. That’s unfortunate and ironic, as the services could prove extremely beneficial in situations where lives are on the line.
To help address the needs of these workloads, many companies turn to open source and transparency. Amazon’s Alexa service is proprietary, but it can work on a Raspberry Pi, which operates on the open source operating system Linux.
Google has gone even further by open sourcing its TensorFlow AI, an open source software library for machine intelligence that can run anywhere. In the enterprise, tools like the Insights analysis engine are being open sourced, and efforts are underway to increase the transparency of the data pipe between customer systems and Insights so customers can know, inspect, and validate what’s being sent back and forth.
Open source and transparency will also be necessary as the internet of things continues to grow. Devices like driverless cars, UAVs, smart city applications, and other autonomous vehicles and systems will need to connect and communicate with each other outside of their own systems.
One vendor’s driverless cars communicating with each other is great, but it would be even better if they could interact with other vendors’ systems, thereby creating a more valuable communications network as illustrated in Metcalfe’s Law. Lightweight, open source messaging systems like MQTT, which enable smart devices to “speak” with each other and various back end AI cloud providers, can help facilitate IoT interoperability.
Finally, the freedom from vendor lock-in cannot be overstated. By standardizing on open source implementations, agencies can free themselves from proprietary shackles and pave the way to add future AI technologies that haven’t been invented yet.
To sum up, AI is for real, and it’s being used today to find better search results, filter spam and provide insights into large sets of data. The key is for the government to work closely with industry on open source and transparency to help agencies experience the benefits the private sector and consumers are already enjoying.